AI In Software Test Automation: The Future Is Here
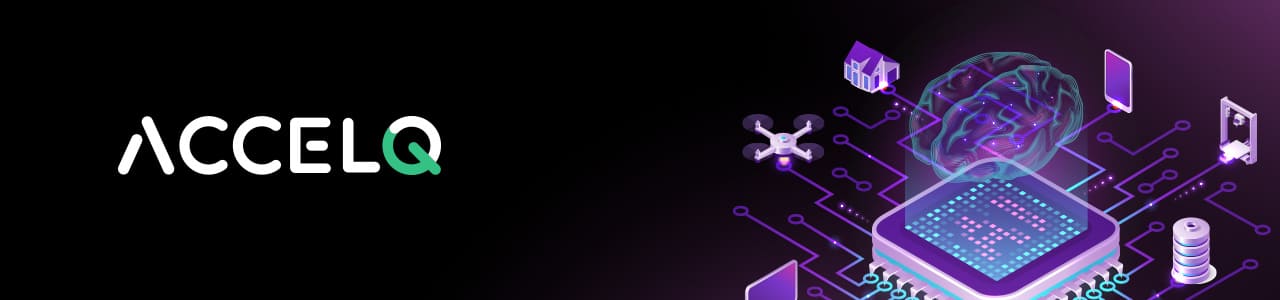
The fusion of Artificial Intelligence (AI) with test automation is a technological advancement. Here, AI reinvents the game rules more than just doing software testing. It has the ability to learn, adapt, and predict, transforming test automation into a more efficient process.
The AI in Test automation increases the chance of uncovering hidden flaws and foreseeing potential pitfalls. Let's explore how AI is changing how we test software and reshaping the future of digital excellence.
Need for Test Automation
Speed and accuracy in software development have become far more than an expectation. Test automation addresses this with repetitive, detailed, and data-intensive testing. It ensures software quality in agile and continuous delivery environments.
What is AI Automated Testing?
AI in test automation became a significant shift in quality assurance. Its ability to learn, adapt, and predict enhances test automation with perfect efficiency and accuracy. Complex algorithms now act as quality detectives, identifying hidden issues and forecasting potential problems. AI automated testing is altering our testing methods and shaping the future of digital innovation.
Using AI for Test Automation?
AI can be integrated into various stages of the testing lifecycle, from test case generation to execution and maintenance. Tools like Selenium for web crawling or AI-based ACCELQ test automation demonstrate the practical applications of AI in automating testing processes.
Do more with Test Automation
Discover more ways to add ‘low-code no-code‘ test automation in your workflows
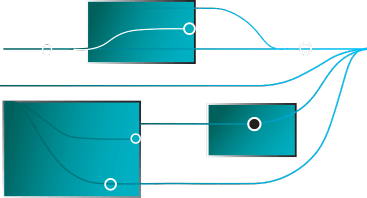
AI vs ML vs DL
Aspect | Artificial Intelligence (AI) | Machine Learning (ML) | Deep Learning (DL) |
---|---|---|---|
Definition | The broad concept of machines carrying out tasks in a smart, human-like manner. It encompasses techniques that enable computers to mimic human intelligence. | A subset of AI involving algorithms that allow software to predict outcomes more accurately without explicit programming. Focuses on systems that learn from data. | A subset of ML involving neural networks that learn from large data sets. Attempts to simulate the human brain’s decision-making process. |
Applications | Used in natural language processing, robotics, expert systems, and more. Examples include voice assistants and autonomous vehicles. | Applied in recommendation systems, spam filtering, predictive text, and more. | Utilized in advanced applications like image and speech recognition, natural language understanding, and autonomous driving. |
Impact on Testing | In test automation, AI analyzes test results, identifies data patterns, and decides which tests to run. | Applied in test automation for predicting likely failure areas, optimizing test cases, and reducing manual test creation effort. | Used in test automation for complex tasks like visual recognition in UI testing, interpreting application visuals more effectively. |
The Role of AI in Test Automation
What is the role of AI in software testing? Here's a more detailed look at its role and impact:
Authoring Unit Test Cases
Gone are the days of intense test script creation. Now, developers and analysts can quickly generate test cases with no code required. AI's statistical power crafts these scripts, ensuring they are fast and thorough. This means keeping pace with rapid code development is now a breeze, leaving behind the old ways of template dependence.
Example:
A team working on a banking app uses AI to automatically generate test cases for new features like fund transfers and account updates. The AI analyzes the app's functions and user interactions to create comprehensive test scripts, significantly reducing the time and effort required for manual scripting.
Faster Test Data Generation
Digital platforms are bombarded with user interactions daily. To mimic this in testing, you need a mountain of data. AI steps in here, adeptly crafting test datasets that mirror real-life scenarios. This isn't just data, it's a reflection of the real world, enhancing the accuracy and reliability of your digital applications before they hit the market.
Example:
An e-commerce platform experiences varied customer behaviors, from browsing products to completing purchases. AI simulates these behaviors, generating diverse test data that reflect different customer profiles and shopping patterns, ensuring the platform is robust under various real-world scenarios.
Powerful UI Validation
When it comes to user experience, every detail counts. Manual UI testing can be subjective, but Machine Learning changes that. It learns what users expect from an interface, applying this knowledge to validate UI designs autonomously. This ensures your digital products look good and meet customer expectations every time.
Example:
A travel booking website uses machine learning to validate its user interface. The AI learns from user feedback and navigation patterns, automatically adjusting the layout and design elements to enhance usability and visual appeal, ensuring a seamless booking experience for users.
Uncover Hidden Defect Patterns
Traditional testing can miss subtle yet critical changes, leading to overlooked defects. AI-powered testing is like a detective sifting through data from countless test cycles. It spots hidden behavioral changes, even minor ones, ensuring every aspect of the application is scrutinized. This meticulous approach guarantees a final product that's functional and flawless.
Example:
In a cloud storage service, AI-driven testing tools analyze historical test data to identify patterns in file upload failures. The AI uncovers a defect related to specific file formats and sizes, which was previously missed, allowing the team to rectify the issue before the next software release.
Conclusion
AI can bring about significant changes in test automation and help enterprises embrace technology innovations at a faster rate. It empowers test professionals and software developers with a more comprehensive and unbiased testing capability. This approach covers both common and exhaustive usage scenarios for the product. It effectively eliminates even the smallest risks from creeping into production-ready code.
Get in touch with us to learn more about autonomous test automation with AI and how your enterprise technology can adopt it for better QA results.
Suma Ganji
Senior Content Writer
Expertly navigating technical and UX writing, she crafts captivating content that hits the mark every time. With a keen SEO understanding, her work consistently resonates with readers while securing prime online visibility. When the day's work ends, you'll find her immersed in literary escapades in her quaint book house.
Discover More
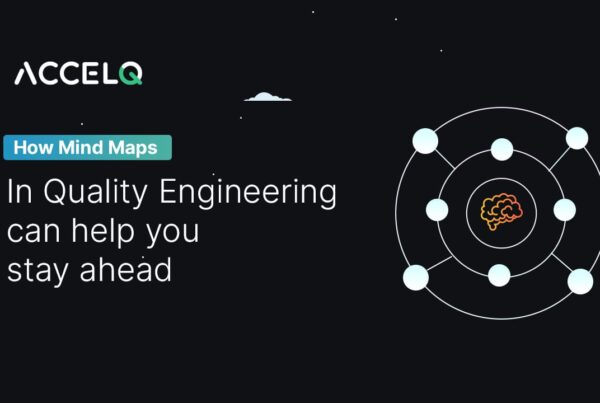
How Mind Maps in Quality Engineering Can Help You Stay Ahead
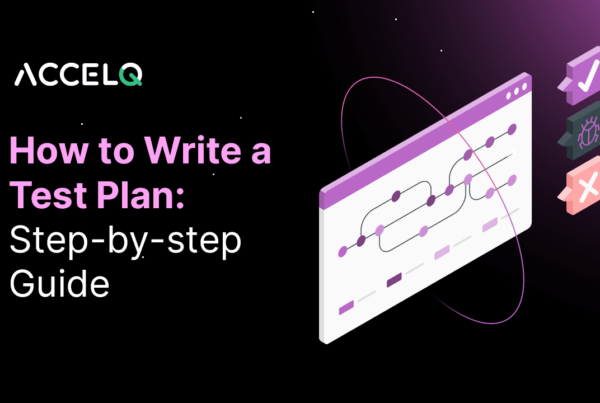